MLOps Solutions for Advanced Machine Learning and AI Efficiency
Our MLOps solutions reduce costs while incorporating operational efficiency into your ML Development. As a MLOps company, our LATAM team focus on what matters most: AI-driven strategies for business outcomes
Why MLOps For Your Business
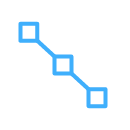
Consistency & Reproducibility
You can confidently replicate successful models and streamline decision-making processes.
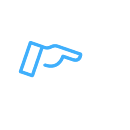
Improved
Collaboration
Break barriers between teams with a seamless flow of information and code.
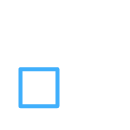
High
Scalability
Automated deployment and scaling for ML models to meet your business needs.
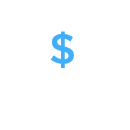
Reduced
Costs
You can detect issues early with automated development pipelines and faster time-to-market
Empower Your Business with our Certified Machine Learning Team.
Our MLOps Solutions to Optimize Processes and Drive Results
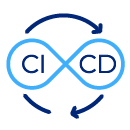
CI/CD automation
We automate the process of building, testing, and deploying your app while maintaining reliability and stability.
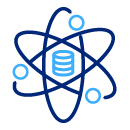
Our data science experts extract actionable insights, uncover hidden patterns, and derive meaningful business intelligence from your data.
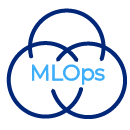
MLOps Integration
We integrate popular ML frameworks and DevOps tools such as Tensorflow, PyTorch, Jenkins, Docker, Git.
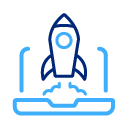
Deployment and Maintenance
we detect issues early so your ML models remain practical and relevant, from initial deployment to ongoing maintenance,
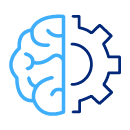
Intelligent Automation
We automate repetitive tasks and workflows, enhancing efficiency to decrease operational costs and minimize manual intervention.
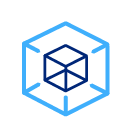
Model Governance
We monitor model performance in real time and set up alerts for drift detection so you can comply with regulatory standards.
Our Clients
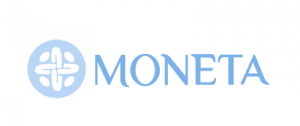
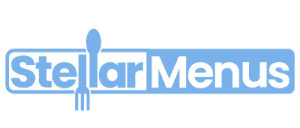
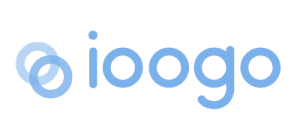
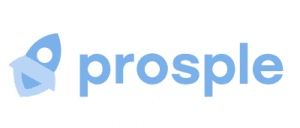
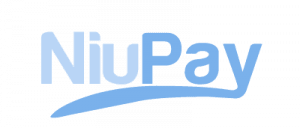
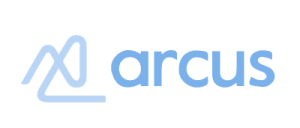
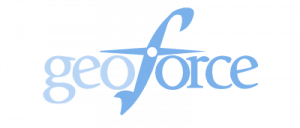
Unlock the Full Potential of your Data-driven Strategies.
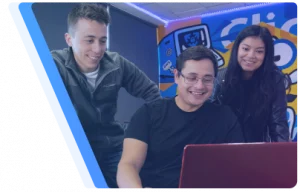
FAQS
Questions About MLOPs Solutions
What do MLOPs solve?
MLOPs integrate machine learning workflows into the DevOps framework while adapting its practices and principles unique to ML development, addressing the challenges associated with operationalizing machine learning, such as model versioning, scalability, reproducibility, and monitoring.
Is MLOPs better than DevOps?
The choice depends on the organizational requirements. If your organization works with machine learning model development and deployment, MLOps is good. On the other hand, DevOps suits organizations that focus on overall software development
What language is best for MLOps solutions?
Commonly used languages in MLOps include Python, due to its popularity in the machine learning community, and languages like Java, Scala, and Go for building scalable and robust production systems.
Which company uses MLOps?
Several companies have adopted MLOps practices to streamline their machine learning workflows. Some notable examples include:
Google, Facebook, Netflix and Airbnb